What Do You Need for Real-Time Data Analysis?
Article Source: Real-Time Analytics
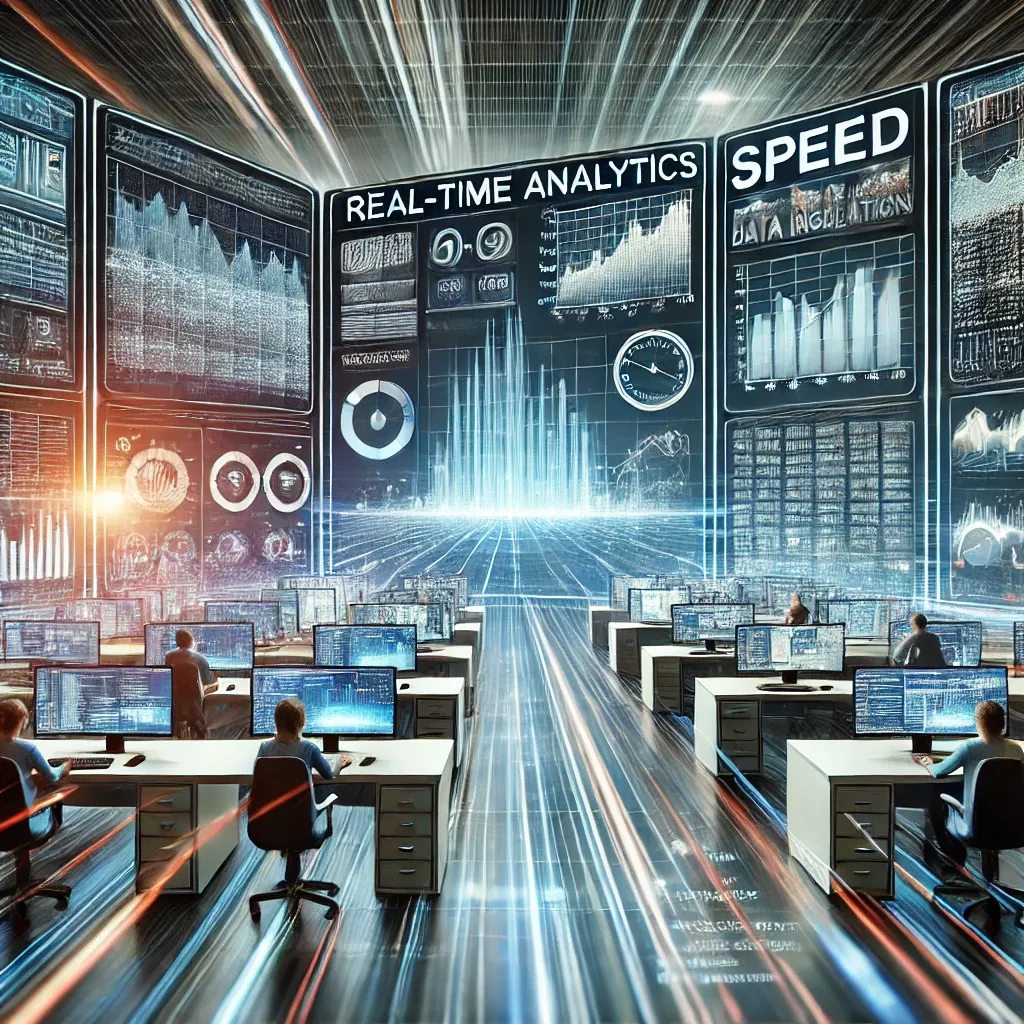
Why You Should Care
In today's fast-paced world, businesses need to make decisions quickly. Real-time analytics helps companies process data instantly, allowing them to react to trends, make informed choices, and stay ahead of the competition. This technology is crucial for industries that rely on up-to-the-minute information, like finance, healthcare, and e-commerce.
Answering the Question… What Do You Need for Real-Time Data Analysis?
Real-time analytics requires high-speed data processing, low latency, and the ability to handle large volumes of data. According to the research, systems must process data in milliseconds to be effective, with the ability to scale up as data demands grow. For example, businesses need systems that can analyze thousands of transactions per second to make timely decisions.
How Was the Study Done?
Researchers examined the infrastructure needed for real-time analytics by analyzing current technologies, including data processing frameworks and hardware capabilities. They reviewed case studies from various industries to understand the challenges and best practices for implementing real-time analytics.
What Was Discovered?
- High-Speed Processing: The study found that real-time analytics systems must be capable of processing data within milliseconds—specifically, in less than 100 milliseconds. This rapid processing is crucial for industries like stock trading, where even a one-second delay can result in significant financial losses.
- Scalability: Scalability is a key requirement, as the data being processed can range from gigabytes to petabytes. Systems need to scale efficiently to handle millions of data points per second, particularly in sectors like e-commerce, where large volumes of customer data are processed simultaneously. For example, the report highlights that top e-commerce platforms often need to process up to 100,000 transactions per second during peak shopping events.
- Low Latency: Low latency is another critical factor, with an ideal target being sub-second latency across the entire data processing pipeline. The research emphasizes that delays of just a few seconds can render data less actionable, particularly in real-time decision-making scenarios such as emergency response or fraud detection.
- Data Integration: The ability to integrate and process diverse data sources is essential. Real-time analytics systems must handle both structured data, such as databases, and unstructured data, such as social media streams. The study found that leading systems can integrate hundreds of data sources, processing and correlating data from various inputs to generate insights within seconds.
- Fault Tolerance: Fault tolerance is crucial for maintaining data flow continuity, especially in systems that cannot afford downtime. The study reveals that real-time analytics platforms often incorporate redundancy and failover mechanisms to ensure at least 99.99% uptime, which is critical in industries like healthcare where system failures could have life-or-death consequences.
- Efficiency and Cost-Effectiveness: The research also discovered that achieving real-time analytics is not just about speed; it’s also about cost. Systems need to be designed to be both energy-efficient and cost-effective, handling massive data loads without incurring prohibitive operational costs. For example, cloud-based analytics solutions were found to reduce costs by 20-30% compared to traditional on-premises setups, primarily due to their flexible scaling and resource management capabilities.
Why Does It Matter?
Real-time analytics is transforming how businesses operate, making it possible to react to changes as they happen. This technology is vital for industries where timing is everything, such as stock trading or emergency services. By understanding and implementing real-time analytics, companies can gain a competitive edge, improve customer experiences, and optimize their operations.
Link to full article: Real-Time Analytics